With massive new surveys like the upcoming Vera Rubin Observatory, which is due to begin full science operations in August 2025, astronomers are worrying about how to cope with the upcoming deluge of data.
Personally, I’m particularly excited about how, among the terabytes of images it will produce every night, we’re going to find the really unusual and unexpected.
One research paper might provide the answer, describing a tool that makes creative use of modern artificial intelligence to guide astronomers to interesting objects.
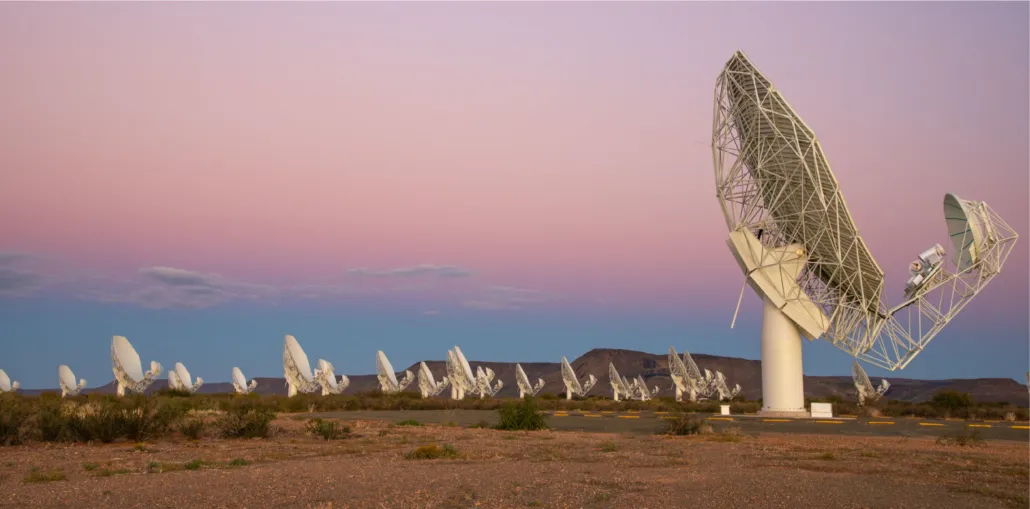
As a test of their method, Michelle Lochner and Lawrence Rudnick set out to explore data from the South African radio telescope MeerKAT.
It spent 6–10 hours staring at each of more than 100 galaxy clusters, together containing more than 6,000 radio galaxies.
How do we decide which galaxies to focus on? That’s where a piece of software that the team call ‘Protege’ comes in.

Help from machine learning
At its heart is a machine-learning routine called BYOL.
BYOL takes a set of images and reduces them to a set of features. We could imagine doing the same trick with a stack of images of animals.
There, it might be useful to have one feature that represents a rectangular shape, for the animal’s body. And maybe something that looks like a leg.
Other features might represent stripes or spots, or the shapes made by trees in the background.
Choose enough features and you could represent any image as a combination of these individual elements.
The magic of routines like BYOL is that you don’t need to define the features yourself, as they’re discovered by the network during a process of training.
Some of the features it chooses might have meanings that make sense to humans, but others may be very abstract.

The crucial thing is that similar galaxies should be represented by similar sets of features, and so thinking like this lets you find similar galaxies – they’re the ones with similar features.
What’s exciting is what happens next. Protege shows a random set of images to the astronomer who is using it, who ranks them on a scale of 1 to 5 based on how interesting they are.
Using this input, Protege guesses what score images with a particular set of features are likely to get and uses this to decide what to show the astronomer for the next round of review.
These too are scored and the process repeats until the astronomer is shown only things they find interesting.
Of course, what is considered ‘interesting’ will vary from astronomer to astronomer, but in the MeerKAT example, Protege does find things its trainers missed – galaxies surrounded by background emission, for example – but it also identifies a fascinating and unexpected set of X-shaped sources.
Some of these seem to be systems where radio jets extend on both sides of the galaxy, but others, with long and sometimes faint ‘wings’ leading away from the central source, are much more confusing.
Even this example study, with ‘just’ 6,000 sources, has produced a set of galaxies we’d love to know more about.
When Protege and its robot friends are set loose on those large datasets that are coming, who knows what we’ll find?
Chris Lintottwas reading Astronomaly Protege: Discovery Through Human–Machine Collaboration by Michelle Lochner and Lawrence Rudnick. Read it online at: arxiv.org/abs/2411.04188.
This article appeared in the January 2025 issue of BBC Sky at Night Magazine