Studying the composition of meteorites provides vital clues about what was happening in the early Solar System and how the planets in it formed.
This is, after all, why astronomers study samples from other worlds.
By far the greatest insights are provided by meteorites, particularly the fallen space rocks that can be collected immediately, before they have a chance to become weathered and contaminated by lying on Earth’s surface.
Over recent years, automated networks of sky-watching cameras have enormously increased the rate at which meteors can be detected while they’re still fireballs falling through Earth’s atmosphere.
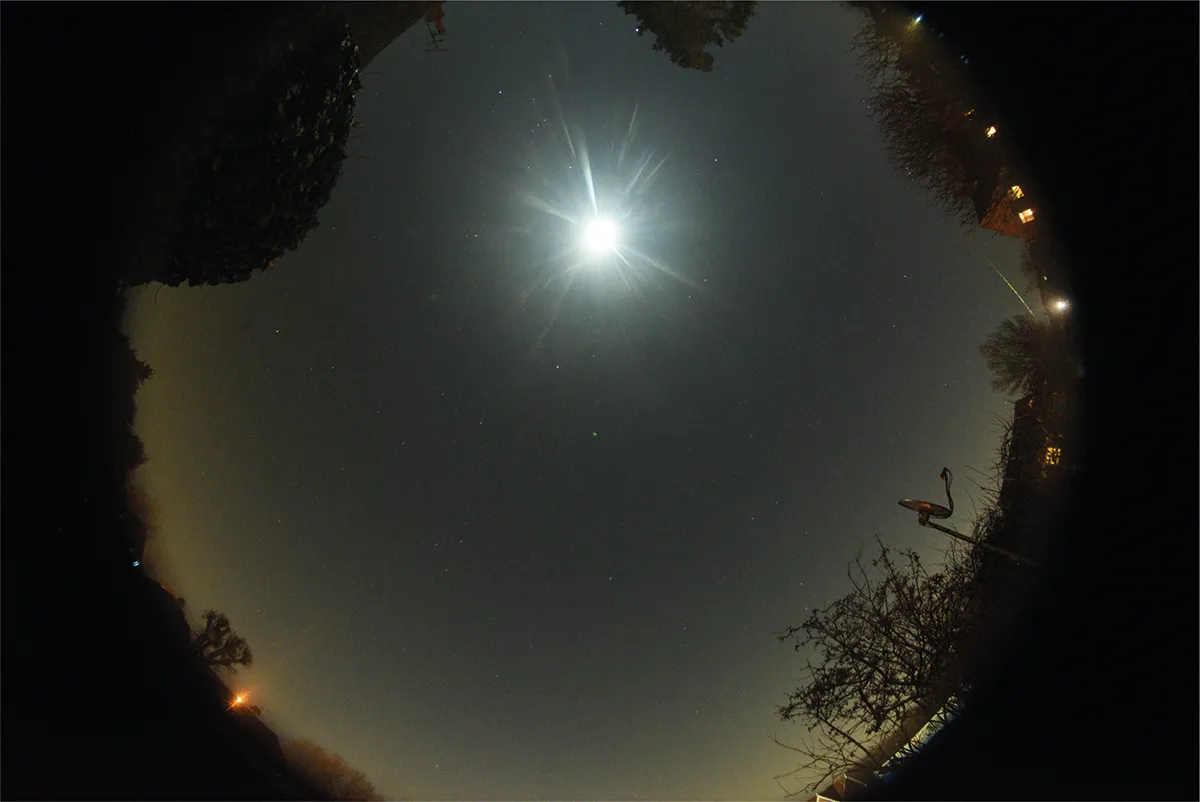
One example is the UK Fireball Network.
Read more about a Staffordshire meteorite hunt sparked by a meteor sighting.
In some cases, the fireball’s trajectory can be extrapolated forwards to predict roughly where the meteorite fragments will land, as well as backwards to determine the orbit of the original meteoroid.
Recovered meteorites with a known point of origin are uniquely useful.
The trouble with finding meteorites

The problem is, even with an observed fall, it can be enormously labour intensive and time consuming to find the small meteorite fragments.
Not all meteorite discoveries are as fortunate as that of the Winchcombe meteorite.
Finding meteorites relies on a team of 5 or more people walking in regularly spaced lines, scouring the ground over several square kilometres. Even then the success rate is low.
But Seamus Anderson and his colleagues at the Space Science and Technology Centre, Curtin University, in Australia, think they have the solution.
Could drones be the answer?
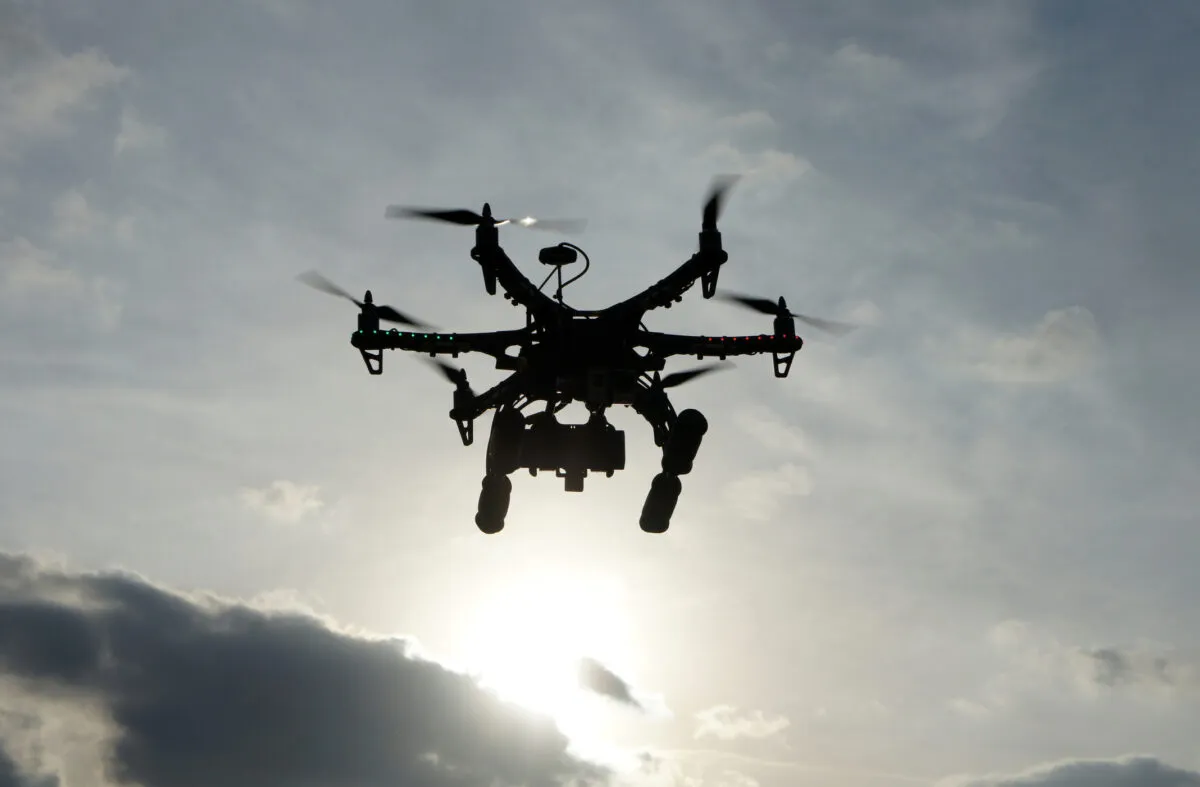
They’ve developed a technique that uses drones to rapidly photograph large areas around the predicted meteorite fall site, and machine learning software to identify potential meteorite candidates in the images.
AI in astronomy is nothing new, but this newly developed system was tested in April 2021.
Two cameras in the Desert Fireball Network in Western Australia detected a bright, three-second fireball and automatically alerted Anderson’s team of scientists.
It was observed down to an altitude of 25km while lancing through the atmosphere at an angle of 64°, and from this trajectory the likely fall line along the ground was calculated.
Anderson and his team spent three days surveying the 5km2 search area, taking tens of thousands of photos with a drone.

After each flight, their computer program, based on a neural network – a simple, artificial brain that had been trained to recognise blackened meteorites lying on the ground – processed the images and highlighted potential fragments.
These meteorite candidates were then remotely checked by the drone, which would return to the specific locations on autopilot and hover just a metre above the ground to take high-resolution images.
Anderson’s team then checked the close-up photos to confirm which ones were most likely to be meteorites and only then visited the specific locations to retrieve the rocks.
Anderson and his team were rewarded with the discovery of a 70g piece of the meteorite, which was found just 50m from the fall line predicted by the fireball tracking.
This is the first time that a ‘fresh’ meteorite has been recovered using such a strategy, and it represents a huge saving in time and effort for scientists.
There’s still room for improvement in the system, though, according to Anderson.
The automated process flagged up a number of false-positives – objects on the ground that weren’t actually meteorites. They included tin cans, bottles and even kangaroos!
Lewis Dartnell was reading Successful Recovery of an Observed Meteorite Fall Using Drones and Machine Learning by Seamus L Anderson et al. Read it online at arxiv.org/abs/2203.01466.